Each of the above tests may be used for two different goals: detection (Table 1) or diagnosis (Table 2) of the disease.5–21
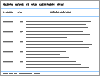 | Table 1 Data Presentation in a Selected Population, Assessing the Detection Capability of a Test. |
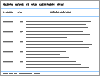 | Table 2 Data Presentation in a Clinical Study Setting in a Target Patient Population Assessing the Diagnostic Capability of a Test. |
Detectability Measures: Technical Characteristics of No Clinical Importance
We use italic lower-case letters in the description of screening in the general population in a 2×2 table,
Table 1. The
sensitivity and
specificity are calculated in samples of persons with (a+c) and without (b+d) the disease in a selected population. In this table, it is not appropriate to include the totals of the “horizontal” axis of test (T) results.
A researcher could determine the detectability of COVID-19 in a study where the prevalence of the disease is artificial. For example, a study may calculate the sensitivity and specificity of a test in 100 persons with a disease (e.g. clinical COVID-19), and 100 persons without the disease. We set the prevalence of COVID-19 in this particular study, artificially, to be 50%.
The sensitivity and specificity are used to describe the technical characteristics of a test. These measures are not useful in the clinical setting, because the prevalence of the disease is different in a true patient population. The sensitivity or specificity could tell us the percentage of the persons with (or without) the disease that would be detected, but we will not know how many patients with COVID-19 (or without it) will be diagnosed correctly. For example, we could know the percentage of persons with the disease that would be quarantined based on detection by a test, but we could not know how many persons would be quarantined. The information on the percentage of detected persons would be meaningful only if we know the prevalence of the disease.
The fraction (percent) of persons with the disease who would not be identified (i.e. the false negative fraction) is fnf = 1 – sensitivity. The fraction (percent) of persons without the disease who would not be identified (i.e. the false positive fraction) is fpf = 1 – specificity.
The Youden index (J) is a summary measure of the goodness of a test. It describes the percent of correct detection (without false negative nor false positive detection). This index is defined as:
J=1-(fpf+fnf)=1-[(1-sensitivity)+(1-specificity)]=sensitivity+specificity-1 | (Eq. 1) |
When J=1, the test is always correct: there are no errors, so fpf + fnf = 0, and the test detects correctly the sickness status.
When J=0, assuming that sensitivity and specificity are of equal importance in determining the expected gain, the test provides no information. In other words, the test is useless if the proportion of errors equals 100%, and fpf + fnf = 1, leading to J=0.
When −1 < J < 0, the test is misleading: its results are negatively associated with a true diagnosis. When J = −1, the test is always misleading.
J can also be interpreted as the difference between the true and false positive fractions.
Since J = sensitivity – fpf, J reflects the excess of the proportion of a positive result among patients with the disease versus patients without the disease. Similarly, J also reflects the excess in the proportion of a negative result among patients without the disease versus patients with the disease. This can also be written as J = specificity – fpf.
J as a difference measure of detectability analogies to a cohort study. Table 1 is analogous to a clinical trial or a cohort study that compares the risk of a disease among those exposed to a risk factor, Rexposed, and the risk among those who are not exposed, Rnon-exposed. The “causative” variable (i.e. the “exposure”) is the fact that a person does or does not have the disease, and the diagnostic test results (positive or negative) are the “outcome” of the disease. The difference in risk between the exposed and non-exposed persons is measured by the risk difference (RD):
RD=Risk difference=Rexposed-Rnon-exposed | (Eq. 2) |
J is analogous to RD.
Therefore, a derived analogy of the well-known measure of the “number needed to treat”, (NNT) = 1/RD, is 1/J. The value 1/J may be interpreted as the number of persons that need to be examined in order to correctly detect by screening (nns) one person with the disease (Table 1) of persons with and without the known disease. The nns could help in estimating the minimum number of tests that has to be applied to persons with known diagnosis of COVID-19 (with or without the disease) in order to detect one person correctly (positive or negative, respectively). It can be useful in assessing a percent of a successful monitoring program (how many of the persons with, or without, the disease will be detected). However, it cannot assess how many persons with or without the disease will be detected, and thus it has no clinical or public health importance, because it cannot be applied to a real population in which we do not know the COVID-19 diagnoses.
Currently, PCR tests have a sensitivity or specificity of approximately 70%–95%, depending on the conditions of the tests.5 For example, the sensitivity of the PCR test using a nasopharyngeal swab is higher than that using a nasal swab, while the specificity of the test is lower using a nasopharyngeal swab. For simplicity, we assume here a 90% sensitivity and 90% specificity for both PCR and serological tests.
Example. Suppose that a population of 1000 travelers is screened for COVID-19, using the PCR test. The test will detect 90% of the persons with COVID-19. These persons will be treated or quarantined. However, the test will not detect 10% of the persons with the disease, i.e. the test will have an fnf of 10%. This would allow 10% of the persons with COVID-19 to continue interacting with their family and the community, with the implied risk of transmitting the disease. Similarly, the test would correctly detect 90% of the persons without COVID-19. These persons would not be quarantined. However the test would incorrectly detect infection in 10% of the uninfected persons, i.e. the test will have an fpf of 10%. This would allow 10% of the persons without COVID-19 to be unjustifiably quarantined. For these data, J would be 0.80. This indicates 80% better information compared to the case where no test is used, or if a useless test with J=0 is used. Note that we know only the test’s ability to detect the percentage, and not the number of persons that would be diagnosed with or without the disease. We know that the test will detect 90% of the persons with (or without) the disease. However, since the prevalence of the disease in the traveler population is not known, we will not know the number of persons with the disease (or without the disease, respectively). The test would not show how many travelers would need to be quarantined, nor how many travelers would not be quarantined because of false negative test results. Based solely on detection measures, it is not possible to plan quarantine facilities or to assess the number of persons with COVID-19 who are wrongly released into the community and continue to infect others. Thus, the detection measures cannot be useful for practical planning of public health measures. By contrast, diagnostic measures, which are explained below, can be used for these purposes.
Diagnostic Measures of Clinical and Public Health Importance
The application of a diagnostic test to a patient (target) population utilizes a 2×2 table (
Table 2). To evaluate the effectiveness of the application of a diagnostic test in the patient population, the investigator first observes the outcome, i.e. the test results, and obtains information about the study factor, i.e. the disease status.
We use upper-case letters to describe screening in the patient population in a 2×2 table, Table 2. It is the data in this table that are of interest to the patient (and the physician) and public health officials, answering the following questions: (1) When the test is positive, what is the probability that the patient has the disease? (answerable by the positive predictive value [PPV]); (2) When the test is negative, what is the probability that the patient does not have the disease? (answerable by the negative predictive value [NPV]).
In this clinical setting, the diagnoses are as yet unknown, and the test is used to diagnose COVID-19 in individuals: the PPV and NPV are an estimate of the test’s ability to diagnose patients accurately in a population (based on the real disease prevalence), i.e. of the fractions of patients who are diagnosed correctly as positive or negative, respectively. The PPV is the fraction (percent) of the positive tests in a given population that will correctly diagnose a COVID-19 patient. Similarly, NPV is the fraction (percent) of negative tests that will correctly diagnose a person who is not infected. The fraction (percent) of persons with a positive test who would not have the disease is the diagnostic false positive fraction (FPF), that is calculable as FPF = 1 − PPV. The fraction (percent) with a negative test result who have the disease and is diagnosed incorrectly as not having the disease is the diagnostic false negative fraction (FNF) calculable as FNF = 1 − NPV.
Both PPV and NPV depend on the proportion of the population that has the disease according to clinical or serological criteria, i.e. the prevalence of the disease. Thus, the PPV and NPV provide insight into the expected accuracy of the positive and the negative test results in a given population, by factoring in the ability of the test to detect the disease and the prevalence of the disease in the population.
Suppose that the same test were used in two different populations: population A with a higher disease prevalence and population B with a lower disease prevalence. Then, the PPV would be higher in population A than in population B, because the number of false positives would be a lower percentage of the total number of positive tests in population A. Similarly, the NPV would be higher in population A than in population B.
Predictive summary index as a summary measure of diagnostic ability of a test in individuals. A summary index, the predictive summary index (PSI, Ψ), is a measure of the additional information given by the test results, beyond the prior knowledge (the prevalence of the disease).21 Note that the information from a positive test result beyond what is already known about the disease prevalence is PPV – Prevalence. Similarly, the information from a negative test result beyond what is already known about the probability of no disease (the prevalence of no disease) is NPV – (1 – Prevalence).
Thus, the overall information, i.e. the gain in certainty obtained after a test is performed, beyond what is already known, can be calculated as a summary measure:
Total gain in certainty=[PPV-Prevalence]+[NPV-(1-Prevalence)]=PPV+NPV-1=PSI | (Eq. 4) |
Alternatively, Ψ is a summary of the information that is not derived from errors, FNF and FPF:
PSI=1-(FPF+FNF)=1-[(1-PPV)+(1-NPV)]=PPV+NPV-1 | (Eq. 5) |
If Ψ=1, the test is always correct: there are no errors, so that FPF + FNF = 0; i.e. the test detects correctly the sickness status. When PSI = 0, PPV + NPV = 1, and the test provides no overall information. In other words, the test is useless if the proportion of errors equals 100%; i.e. when FPF + FNF = 1, PSI = 0. For example, if the test results are random and the probability of both PPV and NPV is 50%, then the test is useless and PSI=0. When −1 < PSI < 0 the test is misleading; i.e. the tests results are negatively associated with the true diagnosis. When Ψ = −1, the test is always misleading.
The PSI can also be interpreted as the gained probability of correct diagnosis information, i.e. the difference between the joint probabilities of correct diagnosis (positive or negative diagnosis) PPV*NPV and the joint probabilities of incorrect diagnosis FPF*FNF:
PPV*NPV-FNF*FPF=PPV*NPV-[(1-PPV)*(1-NPV)]=PPV*NPV-1+NPV+PPV-NPV*PPV=Ψ | (Eq. 6) |
PSI as a difference measure of a diagnostic test. The PSI can be interpreted as the difference between the correct prediction of a disease by the test and a false negative result of the test in the target population.
Thus, PSI reflects the excess in the proportion of infected people in those with a positive result versus the proportion of infected people when the test is (falsely) negative.
Similarly, one can also interpret PSI as
Here, PSI reflects the excess in the proportion of uninfected persons when the test yields a negative result versus the proportion of uninfected people when the test is (falsely) positive.
The NND = 1/PSI is analogous to nns, to estimate the number of patients who need to be examined in the patient population, in order to correctly diagnose one person (see Table 2). For example, this can be the number of people who would have to undergo a PCR test to correctly diagnose one person. This measure may be abbreviated as the “number needed to diagnose” (NND). This information has public health importance. It also enables planning of test services to a specific population, based on the prevalence of the disease in this population as well as on the technical characteristics of the test.